Putting an end to the AI cyber responsibility turf wars – Greg Otto
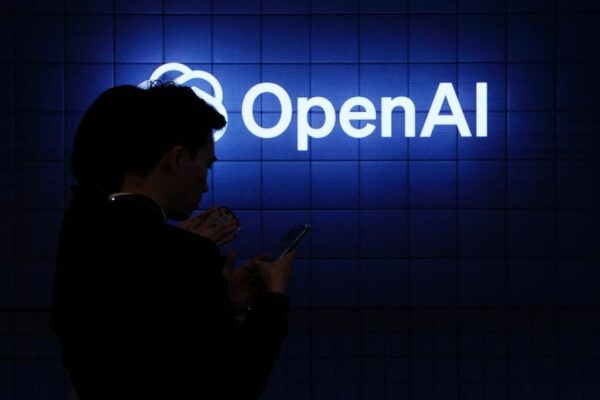
– [[{“value”:”
Since the launch of ChatGPT in November 2022, AI regulation has been hotly debated. Despite the looming cybersecurity risks that generative AI models and large language models (LLMs) pose, regulators have instead been locked in conversations on ethics and social responsibility, leaving potentially catastrophic vulnerabilities by the wayside.
This is not for lack of risk comprehension; the industry has brought forth proof of issues, including data poisoning, model evasion, malware, misuse and more. This recognition has set some planning in action, like the Biden administration’s artificial intelligence executive order. The problem here is that the “check-the-box” measures within an executive order fall short, and there is dispute over who is responsible for determining more effective solutions.
From the perspective of federal leaders, private industry should take the brunt of cybersecurity responsibility as signaled by efforts like the Cybersecurity and Infrastructure Security Agency’s secure-by-design pledge. On the other hand, private industry has looked to legislators to establish goalposts for cybersecurity. Then there are the end-users, who are eager to adopt AI models, but are entangled in security turf wars or ownership confusion between traditional CISOs and CTOs, and emerging titles like chief AI officer and chief data officer.
There is a pivotal point of view that is missing in all this discourse: security is a mindset. No technology tool is without flaws, and making AI models secure is a continual, shared responsibility for all. There is necessary due diligence between public, private and end-users to ensure strong cybersecurity measures however they can, from drafting mandates, to developing the models, to introducing the technology to products and workflows.
How government leaders must activate
For the regulators at the top of this supply chain, effective AI cybersecurity legislation is a must. Comprehensive legislation can elevate conversations around the critical operational aspects of AI to the appropriate level of attention. The rapid proliferation of this technology across industries makes cybersecurity a significant, present concern.
These efforts do not start from ground zero. Regulators should consider following previously laid groundwork, such as the EU AI Act, and the precedents set around other cybersecurity threats. For example, measures like the Software Bill of Materials (SBOM) mandate have created a path for vendors to provide transparent, secure software. The same could one day be said for an AI Bill of Materials (ABOM) mandate.
How AI model developers must activate
While federal regulation in the U.S. may be slower to materialize, there is a lot AI providers can do in the meantime. In the race to AI, many have been quick to develop AI offerings without appropriate focus on the security implications. But attacks against AI systems are not conceptual. There are real, credible threats that can be uniquely defended against with proper planning.
Security committees and oversight controls for even the largest and most well-funded of models did not start to emerge until long after their public release. For the better of the nation, prioritizing speed-to-market over security cannot continue to be the model for innovation.
The major LLM providers have a responsibility to build these models with security best practices in mind. If an AI model were a building, these providers would be the company constructing the building to code. As regulations emerge, these “codes” will get more defined, but until then, security must be emphasized at every step of development with oversight from dedicated experts before models are shared more widely.
How organizations leveraging AI models must activate
As for the organizations embedding these AI models within their products, it is critical they never trust a model straight out of the box, whether they are leveraging a third-party-hosted model or an open-source one. After evaluating the robustness of an AI model’s security, these organizations have an additional responsibility to adopt more safeguards within their own implementations. Following the building analogy, they now need the fire alarms, smoke detectors and evacuation routes.
Organizations seeking to use AI should adopt and utilize a collection of controls, ranging from AI risk and vulnerability assessments to red-teaming AI models, to help identify, characterize, and measure risk. These controls are important in the new era of AI and LLMs, as we can no longer rely on conventional cybersecurity practices for AI systems. Most existing tech stacks are not equipped for AI security, nor do current compliance programs sufficiently address AI models or procurement processes. In short, traditional cybersecurity practices will need to be revisited and refreshed.
Putting all the controls in place — together
For now, approaching AI models with a security-first mindset is the best plan for every member of the supply chain. This is necessary for the nation to securely take advantage of the improved outcomes, speed, and cost effectiveness that AI can provide across government and industry. It is that value and ubiquity that demands the urgency of action.
By pushing forth without acknowledging and addressing the known risks of these models, we are willfully increasing the blast radius for cyber threat actors. AI cybersecurity is a shared responsibility, and there is a role to play for all.
Matt Keating is head of AI security at Booz Allen Hamilton. Malcolm Harkins is chief security & trust officer at HiddenLayer.
The post Putting an end to the AI cyber responsibility turf wars appeared first on CyberScoop.
“}]] – Read More – CyberScoop